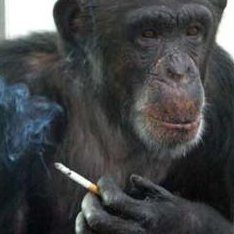

Yup. Google consistently gets rid of features or services that it deems unprofitable. And that’s fine, really - as long as you don’t pretend that you’re doing it for the users.
To be fair in modern phones there are some features that if removed would make the user experience better.
I hear ya - for example, the SIM toolkit being able to send you pop-ups (phone providers use that to spam the users).
It’s hard for Google to claim that they’re focusing resources (e.g. dev time), given the list of features being removed. As one of the HN comments said, quite a few of them “seem to fall under the umbrella of “features that actually make the assistant an assistant”/connecting the assistant to other apps”. In other words, integration - that’s core functionality for an assistant and they likely know it.